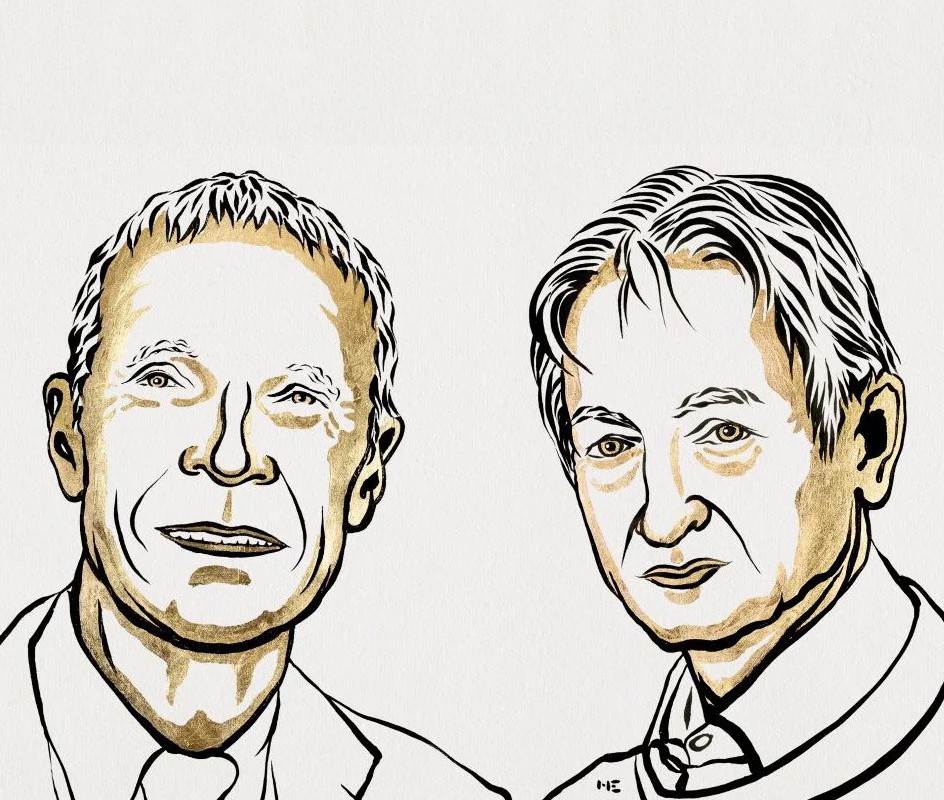
The Royal Swedish Academy of Sciences has awarded the 2024 Nobel Prize in Physics to John J. Hopfield of Princeton University and Geoffrey E. Hinton of the University of Toronto for “foundational discoveries and inventions that enable machine learning with artificial neural networks”. This historic recognition marks the first time that machine learning (ML) research has received the ultimate accolade in academia. It comes just a couple of years after the initial release of ChatGPT (November 30, 2022), a powerful model that revolutionized our daily lives and ways of thinking.
ML allow computers to learn from real-world data, much like humans learn by experiencing their surroundings. Imagine you have a vast collection of photos of cats and dogs—this is real-world data. By feeding these images into an ML model, it can learn to distinguish between cats and dogs based on features like fur patterns and ear shapes. This type of ML often relies on artificial neural networks, class of models inspired by the structure of the human brain. These networks consist of layers of interconnected nodes (neurons) that process data in a way that similar to how our brains work. As data passes through these layers, each one extracts increasingly complex features, enabling neural networks to excel in tasks like image recognition, natural language processing, and even playing complex games.
John J. Hopfield developed the Hopfield network, a type of artificial neural network that allows for the storage and recall of patterns—similar to how our brains remember images or sounds. His network can reconstruct complete images from partial or noisy inputs by adjusting the connections between its nodes to minimize errors. Geoffrey E. Hinton, born in 1947 in London, UK, further advanced this work with the Boltzmann machine, inspired by Hopfield networks. This machine learns to recognize patterns in data by adjusting its connections to find the most likely ones, making it useful for tasks like image classification and generating new examples from learned patterns.
Representation of Hopfield’s network (left) and Hinton’s Boltzmann machine (right). Image taken from https://www.nobelprize.org/prizes/physics/2024/popular-information.
What exciting developments await us in the future? Would it surprise you to learn that this article was written by artificial intelligence? Can you even tell? As we celebrate this monumental achievement for the machine learning community, we at CICECO are both excited and optimistic about the future. We are actively involved in applying ML to model new material properties, such as predicting the efficiency of solar concentrators for clean energy harvesting [1,2] and studying physicochemical properties [3–5].
Recently, CICECO hosted a group of global experts to discuss the future of artificial intelligence. If you missed it, you can catch up here: https://www.ciceco.ua.pt/?language=eng&menu=628&tabela=geral
https://www.youtube.com/watch?v=pdimP0rVFpg&list=PLl57eQK6k-zVlsiUZn5VH4K0wCfAw60Ns
[1] P.S. André, L.M.S. Dias, S.F.H. Correia, A.N. Carneiro Neto, R.A.S. Ferreira, Artificial neural networks for predicting optical conversion efficiency in luminescent solar concentrators, Sol. Energy. 268 (2024) 112290. https://doi.org/10.1016/j.solener.2023.112290.
[2] R.A.S. Ferreira, S.F.H. Correia, L. Fu, P. Georgieva, M. Antunes, P.S. André, Predicting the efficiency of luminescent solar concentrators for solar energy harvesting using machine learning, Sci. Rep. 14 (2024) 4160. https://doi.org/10.1038/s41598-024-54657-x.
[3] D.O. Abranches, Y. Zhang, E.J. Maginn, Y.J. Colón, Sigma profiles in deep learning: towards a universal molecular descriptor, Chem. Commun. 58 (2022) 5630–5633. https://doi.org/10.1039/D2CC01549H.
[4] D.O. Abranches, E.J. Maginn, Y.J. Colón, Boosting Graph Neural Networks with Molecular Mechanics: A Case Study of Sigma Profile Prediction, J. Chem. Theory Comput. 19 (2023) 9318–9328. https://doi.org/10.1021/acs.jctc.3c01003.
[5] D.O. Abranches, E.J. Maginn, Y.J. Colón, Stochastic machine learning via sigma profiles to build a digital chemical space, Proc. Natl. Acad. Sci. 121 (2024). https://doi.org/10.1073/pnas.2404676121.
This specific text is authored by Dinis Abranches (Department of Chemistry and CICECO - Institute of Materials of Aveiro, University of Aveiro) and Albano Neto (Department of Physics and CICECO - Institute of Materials of Aveiro, University of Aveiro).
Related Articles
We use cookies for marketing activities and to offer you a better experience. By clicking “Accept Cookies” you agree with our cookie policy. Read about how we use cookies by clicking "Privacy and Cookie Policy".